Overview
This guide provides information on integrating external data into the EMERSE application, as well as basics about the indexing process. In order for EMERSE to search and highlight documents, they must be submitted to the Solr instance to which EMERSE is attached. Additionally, patients who have indexed documents need their corresponding demographics loaded into EMERSE’s Patient
table in the database. Optionally, research study and user (personnel) information can be loaded into a few EMERSE database tables that will allow EMERSE to check that authenticated users are research personnel for approved studies, which is used for determining research access and populating the audit tables at the time of login.
Data integration and indexing are tightly linked, which is why they are discussed together in this guide. Not covered in depth is Solr itself, as this is already well documented within the Apache Solr project itself. Note that knowledge of Solr will be crucial to indexing for EMERSE.
Integrating Documents
The EMERSE application uses Solr to search, highlight, and display documents. This section provides possible approaches for submitting documents to Solr for indexing, as well as information about conforming your documents to the Solr schema that EMERSE requires.
When setting up a new system, it’s best to figure out what fields should exist in Solr, alter Solr’s managed-schema to reflect that, then set up EMERSE’s fields.
Don’t modify managed-schema or other Solr config files while Solr is running. Additionally, altering managed-schema will require a re-index as Solr doesn’t migrate already indexed data so that it reflects your changes to the schema.
|
Documents in Solr
A document is an object that associates values with fields. Each field must hold the same kind of value, whether its a string, number, or date. Solr also bundles up information about how to index the value of the field with the type of the field. These field types and the fields themselves are described in the managed-schema
file in conf
directory. There is one for the patient index and one for the documents index. Here are the basic field types that come in the demo system:
<fieldType name="date" class="solr.DatePointField"
sortMissingLast="true" uninvertible="false" docValues="true"/>
<fieldType name="long" class="solr.LongPointField"
sortMissingLast="true" uninvertible="false" docValues="true"/>
<fieldType name="int" class="solr.IntPointField"
sortMissingLast="true" uninvertible="false" docValues="true"/>
<fieldType name="string" class="solr.StrField"
sortMissingLast="true" uninvertible="false" docValues="true"/>
<fieldType name="boolean" class="solr.BoolField"
sortMissingLast="true" uninvertible="false" docValues="true"/>
<fieldType name="text_cs" class="solr.TextField"
sortMissingLast="true" uninvertible="false" docValues="true"
>
<analyzer>
<tokenizer name="standard"/>
</analyzer>
</fieldType>
<fieldType name="text_ci" class="solr.TextField"
sortMissingLast="true" uninvertible="false" docValues="true"
>
<analyzer>
<tokenizer name="standard"/>
<filter name="lowercase"/>
</analyzer>
</fieldType>
You can read up on types in Solr in the Solr documentation. There are other types you could possibly use, but EMERSE is only really tested with these. |
These field types index the entire value of the field as a single value. So, for instance, if a value of a string
field describing the clinician is "David Hanauer" then only a search for exactly that string will find the document; a search for "David" or even "david" with lower case will not locate the document. This is typically fine, since fields describing metadata such as clinician will be entered in by users via filters, where we list the actual values and let users select between them.
However, for the main body text, you will need to use a different type that tokenizes by words, along with adding additional NLP tokens for use in EMERSE. The type used in the demo system looks like:
<fieldType name="text_with_header_post_analysis" class="org.emerse.solr.TextWithHeaderFieldType"
termPositions="true" termVectors="true" termOffsets="true" termPayloads="true"
>
<analyzer type="index">
<charFilter class="solr.HTMLStripCharFilterFactory"/>
<tokenizer class="org.emerse.opennlp.OpenNLPTokenizerFactory"
tokenizerModel="nlp/models/opennlp-en-ud-ewt-tokens-1.0-1.9.3.bin"
sentenceModel="nlp/models/sd-med-model.zip"
hasHeader="true"
usingLuceneTokenizer="true"
/>
<filter class="org.emerse.ctakes.CTAKESFilterFactory"
excludeTypes="nlp/pos_exclude.txt"
prefix="CUI"
dictionary="nlp/dictionary/custom.script"
longestMatch="false"
/>
<filter class="org.emerse.nlp.TaggerFilterFactory"
addTokenPrefix="false"
delimiters="nlp/delimiters.txt"
negations="nlp/negations.txt"
ignore="nlp/ignore.txt"
certainty="nlp/probability_resource_patterns.txt"
family="nlp/family_history_resource_patterns_A.txt"
familyTemplates="nlp/family_history_resource_patterns_B.txt"
familyMembers="nlp/family_history_resource_patterns_C.txt"
tokenizerModel="nlp/models/opennlp-en-ud-ewt-tokens-1.0-1.9.3.bin"
/>
<filter class="org.emerse.nlp.PostAnalysisFilterFactory"/>
</analyzer>
<analyzer type="query">
<charFilter class="solr.HTMLStripCharFilterFactory"/>
<tokenizer class="org.apache.lucene.analysis.standard.StandardTokenizerFactory"/>
</analyzer>
</fieldType>
More information on exactly what is going on in this type can be found in the NLP Guide. Once you understand these types, you can decide what fields your documents should have. The demo system’s field are defined like:
<uniqueKey>ID</uniqueKey>
<field name="ID" type="string" required="true"/>
<field name="SOURCE" type="string"/>
<field name="MRN" type="string"/>
<field name="CSN" type="string"/>
<field name="ENCOUNTER_DATE" type="date"/>
<field name="DOC_TYPE" type="string" default="unknown"/>
<field name="RPT_TEXT" type="text_with_header_post_analysis"/>
<field name="NLP_HEADER" type="string" indexed="false"/>
<field name="CASE_ACCN_NBR" type="string"/>
<field name="ADMIT_DATE" type="date"/>
<field name="CLINICIAN" type="string" default="unknown"/>
<field name="DEPT" type="string" default="unknown"/>
<field name="INDEX_DATE" type="date"/>
<field name="LAST_UPDATED" type="date"/>
<field name="RPT_DATE" type="date"/>
<field name="RPT_ID" type="string"/>
<field name="SVC" type="string" default="unknown"/>
<field name="CATEGORY" type="string" multiValued="true"/>
<field name="AGE_DAYS" type="int"/>
<field name="AGE_MONTHS" type="int"/>
Each Solr document must be uniquely identified by the value of a single field, called the unique key or ID. The <uniqueKey>
element defines what field that is. Typically we keep this as ID. When you submit a document to the index, it will replace any document that was previously indexed that has the same ID. In this way, you can "update" a document.
A field can also be multi-valued by setting multiValued="true"
on the field’s element. A multi-valued field contains an array or list of values as its value instead of a single one. Each value is indexed independently, and the document can be retrieved by by any of them; you cannot search for a document that contains a particular list of values in a multi-valued field. For instance, if clinician was a multi-valued string field with the value ["David Hanauer", "John Smith"]
you could locate the document by searching by David Hanauer
or John Smith
but not David
, Smith
or David Hanauer John Smith
. It’s not possible to search for a list of values in Solr.
You can set a default value for a field, which is used when no value is given for the field in a document. By default, if a field has no value, it cannot be found by any search on that field. This isn’t necessarily a problem, but if some documents don’t have a value for a given field and there is a filter setup in EMERSE on that field, then not all documents will be represented in the choices for that filter in the UI, which can be confusing for users or administrators. It can also result in users missing documents when doing their work. For instance, if a user was looking for documents from a certain department, they would select that department in a filter. However, if some documents don’t have a department perhaps because of some data-quality issue, users will not be able to find those documents with their search, unless they choose to remove their filter on department. If instead documents got a default value of "unknown" on department, then "unknown" would be a filter option, and users could search for both their target department, and the "unknown" department.
Finally, the document text field (RPT_TEXT
in the demo system) uses a custom type we built to do NLP. This field expects the text of the document to be prefixed with something called the NLP header. See the NLP Guide for more info, but for indexing documents using the default configuration as in the demo system, you should prepend a line with RU1FUlNFX0g=1|5|
to HTML documents, or a line with RU1FUlNFX0g=2|5|
to non-HTML documents. You should also include this prepended line as the value of the NLP_HEADER field.
Whereas the fields of the documents index are highly customizable, the patient index should have exactly the schema defined in the demo indexes; they should not be changed.
Documents in EMERSE
EMERSE models documents in a very similar fashion as Solr, as an association of fields to values. However, EMERSE partitions the documents in Solr into groups called sources. A document belongs to the source named in the SOURCE
field of that document. Then, EMERSE allows a field to be specific to a single source, or general to all sources.
You can view the fields defined in EMERSE in the Fields
tab in the admin app. Each field has a few attributes:
-
a label, which is the value that will be shown to users of EMERSE
-
a Solr field the EMERSE field is mapped to
-
a source, which determines if it’s specific to a source, or general to all sources
-
a type, which in EMERSE is TEXT, DATE, or NUMBER. Typically EMERSE can look at the underlying Solr type and uniquely determine the appropriate type from that, so often there is only one option here
-
a filter UI, which if set, will allow users to set filter on the field
-
display settings related to where if anywhere the value of the field should be shown to users to help describe documents, such as in the document list, or a single-document view
Finally, each field can defined a field value grouping. If a grouping is defined, then each value in Solr can be placed into zero, one, or more groups. Each group is defined only by its label. The group labels can then be shown to users instead of the underyling values in Solr. This allows you to store very short codes in Solr, but provide nice names in the EMERSE UI. Similarly, it allows you to put multiple Solr values in the same group so they are presented the same to user. This can be useful if you want to have a more coarse grouping of documents than the underlying values indexed into Solr, or if say, the codes have changed over the years and you want a consistent set of labels for users to choose from.
There is no rule that each Solr field is used by only a single EMERSE field; you can have multiple EMERSE fields be mapped to the same Solr field. Together with thou value grouping feature, this allows you to present multiple levels of hierarchy from the values of a single field. For instance, if you have a field that stores the lab in Solr, you can create two fields in EMERSE, one what groups labs into departments, and one that remains at the granularity of labs. It can also de-couple compound information. For instance, if you have a field whose values contain information on both what body part was imaged and what type of imaging was done, you can define two fields in EMERSE that map to that single value in Solr, and set up the groups to be either the imaging types, or the body parts.
Though the field value grouping system allows these flexible features, EMERSE ultimately must query Solr using the values stored in Solr. That means, if a user sets a single field value group as a filter, EMERSE will end up querying Solr with all the values in that group. If that is a lot, it will end up being a very large and slow query to run. Thus, these features are mainly intended to allow flexibility in customizing the system after data has been indexed, but when planning a system, it’s best to break down documents in every way you think users will need it, keeping the queries as small as possible so they are as fast as possible.
Date at-time-of-document Fields
There are two Filter UIs you can set on a field which need more explanation in particular: AGE_DAYS
and AGE_MONTHS
. These filter UIs are only available on numeric-valued fields, and are meant to be used to filter on the age the patient was at the time of the encounter the document pertains to. These fields should have declarations in Solr with the int
type, like below:
managed-schema
<?xml version="1.0" encoding="UTF-8"?>
<schema name="example" version="1.5">
<field name="AGE_DAYS" type="int"/>
<field name="AGE_MONTHS" type="int"/>
</schema>
Once set, you can set their type to NUMERIC
in the EMERSE admin UI, and set their Filter UI accordingly too.
The tough part about these fields is loading the correct data into them, as part of the ETL process. They should contain a count of days or months between the birth date of the patient, and the encounter date of the document. For instance, if the birth date of the patient is 2025-04-12
and the encounter date is 2025-05-20
, then AGE_DAYS
should be 38, and AGE_MONTHS
should be one. If the encounter date was 2025-05-11
, they would be just a day shy of a month old, and so AGE_MONTHS
would get 0
. Between these two fields, we can filter on the age of the aptient at the time of the document by days, weeks, months, and years since there are always 7 days in a week, and always 12 months in a year.
Here is some Java code should correctly do the calculation:
class AgeCalculation
{
private static final short[] LEAP_YEAR;
private static final short[] NORMAL_YEAR;
static
{
LEAP_YEAR = new short[]{0, 31, 29, 31, 30, 31, 30, 31, 31, 30, 31, 30, 31};
NORMAL_YEAR = new short[]{0, 31, 28, 31, 30, 31, 30, 31, 31, 30, 31, 30, 31};
short c = 0;
for (int i = 0; i < LEAP_YEAR.length; i++)
{
c += LEAP_YEAR[i];
LEAP_YEAR[i] = c;
}
c = 0;
for (int i = 0; i < NORMAL_YEAR.length; i++)
{
c += NORMAL_YEAR[i];
NORMAL_YEAR[i] = c;
}
// So, NORMAL_YEAR[m] is number of days in the year prior
// to the first of the zero-indexed month m
// Eg, NORMAL_YEAR[FEBRUARY = 1] = 31, since there are 31 days before Feb 1st.
// So, NORMAL_YEAR[MARCH] = 59
}
public static int[] age(GregorianCalendar birth, GregorianCalendar enc)
{
int ageInMonths;
int ageInDays;
// Java month numbers start from zero. Eg, January = 0.
{
var years = enc.get(YEAR) - birth.get(YEAR);
var months = enc.get(MONTH) - birth.get(MONTH);
var days = enc.get(DAY_OF_MONTH) - birth.get(DAY_OF_MONTH);
ageInMonths = years * 12 + months;
if (days < 0) ageInMonths--;
}
{
var birthYear = birth.get(YEAR) % 4 == 0 ? LEAP_YEAR : NORMAL_YEAR;
var encYear = enc.get(YEAR) % 4 == 0 ? LEAP_YEAR : NORMAL_YEAR;
var years = Math.max(0, enc.get(YEAR) - birth.get(YEAR) - 1);
ageInDays =
(years * 365 + years / 4) +
(enc.get(DAY_OF_MONTH) + encYear[enc.get(MONTH)]) +
(birthYear[12] - birthYear[birth.get(MONTH)] - birth.get(DAY_OF_MONTH));
if (enc.get(YEAR) == birth.get(YEAR)) ageInDays -= birthYear[12];
}
return new int[]{ageInMonths, ageInDays};
}
}
Of course, your language may make it much easier. For instance, also in Java, you can just do birth.until(enc, DAYS)
to get the age in days.
Once you can calculate the age in days and months, you only need to integrate it into your ETL jobs. This will depend heavily on how you implement those, but notice in particular that this calculation can’t be done when given only the content of the document you would otherwise index into Solr, since such documents (usually) don’t contain the birth date of the patient. This is easy fix in database systems where you only need to join with another table to do the calculation.
Special Fields in EMERSE
EMERSE a number of general fields that are required. These are listed as "special roles" in the UI. (This only appears for fields listed in the general section.) A single field can play multiple special roles, but typically different fields do. Fields that place a special role generally should have the string
type in Solr, but with some obvious exceptions, as listed below.
CLINICAL_DATE
-
the date of the patient encounter or other medically relevant date. This should be a date type in Solr.
DOC_CONTEXT
-
the field used to title patient snippets in all-patient search. Often this is something like the document type.
MRN
-
the patient’s unique identifier, grouping documents by patient.
PATIENT_ENCOUNTER_ID
-
the patient encounter ID, which links multiple documnets to a single visit from the patient. This is role is optional.
RPT_ID
-
a unique identifier for the document across all sources. This should be the
ID
field in Solr, or whatever is marked as<uniqueKey>
in themanaged-schema
file. RPT_TEXT
-
the field that contains the document text.
SOURCE
-
the field that tells what source the document is from. The values of this field are the sources of documents.
One field in Solr must be present, since it’s used by Solr, called _version_
. It’s definition should be:
<field name="_version_" type="long"/>
<fieldType name="long" class="solr.LongPointField" docValues="true" invertible="false"/>
Extra care must be taken to ensure that the Solr field with special role RPT_ID (henceforth called its ID) is truly unique across all sources. It is often the case that document from different sources will have the same ID. If you submit a document to Solr that has the same ID as one already in Solr, it overwrites said document, thus at most one of the documents will appear in Solr. To solve this, it is best to assign a "code" to each source, where no source’s code is a prefix of any other source’s code, and prefix each document’s ID with the source’s code. For instance, if you have two sources, and you code them as A and B , then an ID 123 present is both sources will be sent to solr as A123 if the document is from source A, and B123 if its from source B. Codes surgery and surgery_er would not work in general, since surgery is a prefix of surgery_er so that (for instance) a document with ID _er123 in source surgery and a document with ID 123 in source surgery_er would both become surgery_er123 , and thus overwrite one another in Solr. Obviously, if you can guarantee no ID in surgery starts with _er then you should be fine.
|
Technical approaches for Solr indexing
Getting documents from source systems will vary considerably at each site and will depend on multiple factors including the number of different sources, how the documents are stored, how they are formatted, the type of access or connections available, etc.
Three high level approaches are briefly described here, with details in additional sections that follow:
- Custom code
-
We have found this to be the ideal approach because of it’s speed, multi-threaded capabilities, and flexibility. Client libraries are available for most common programming languages. See section, below: Indexing Programmatically
- Solr Data Import Handler (DIH)
-
The Solr DIH ships with Solr and is relatively easy to use. It may be a good approach for getting started and learning Solr. However, while the DIH is useful to understand how the retrieval/indexing process works, the DIH is slow compared to other methods and is not multi-threaded so we do not recommend it for large-scale implementations. See: Indexing using Solr DIH (Data Import Handler)
- Data integration/ETL tools
-
Documents can also be presented to Solr using non-developer tools such as Pentaho Data Integration (PDI), which is free and open source, and is used on our demonstration EMERSE Virtual Machine for loading/indexing data. See: Indexing with ETL tools
Indexing Programmatically
We have developed an indexing application to pull data from our databases and push them into Solr via Solr’s JSON update handler endpoints. This is not part of EMERSE itself, but part of what we call the "data pipeline" to EMERSE. The data pipeline also includes additional transformations before the documents reach the database such as converting documents in RTF format to HTML format which is ideal for displaying in EMERSE.
Though we directly index in our code, it is possible to use a Solr client library to help.
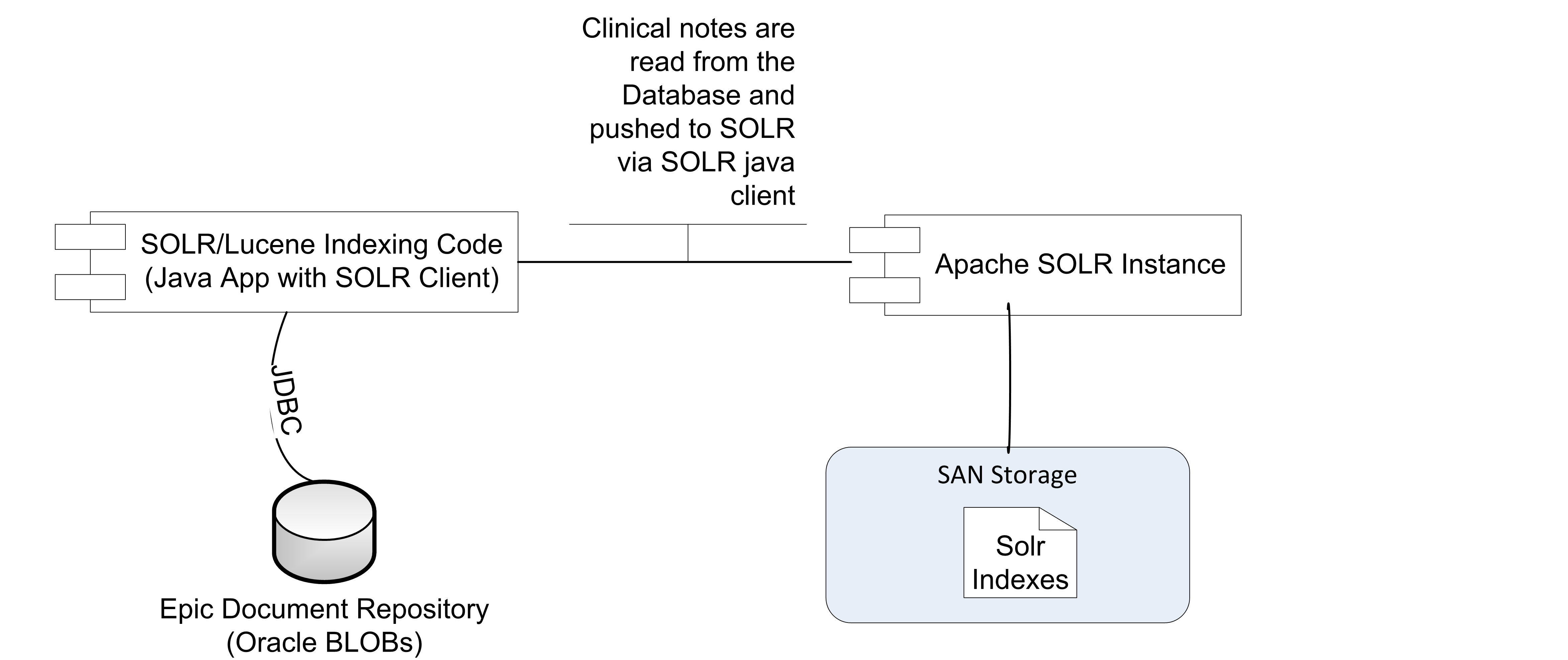
It’s best to send mulitple documents to Solr in a single update request. This can be done by simply sending a JSON array of documents instead of a single document in the request body. You can also send documents to Solr in multiple threads simultaneously.
On the other hand, it is possible to send documents so fast that Solr cannot process them, returning an error. If you see such an error, it’s best to simply make the request again several times until it’s successful, waiting a second or more between to give Solr some time to catch up. The below Java code shows how this code be done with a simple loop. (See the indexDocument method.)
Java Example
Below is the source of a java program which can index a hierarchy of files. This example does not use SolrJ, mainly to keep it is as simple to run as possible. This file assumes Solr is accessible at http://localhost:8983/solr
, and that you have the demo system’s configuration for field names and the index name. For the specific example in the code below, you would also likely need to include the name of the Solr index (also called the core or collection name) to which you are sending the documents, so the final URL may look something like http://localhost:8983/solr/documents
. But you can also add the collection name in the Solr API and leave it as just /solr
at the end of the URL.
To run it, simply copy and paste it into a file named Index.java
, then do:
$ java Index.java
It’ll give more instructions on how it should be run.
import java.io.*;
import java.net.HttpURLConnection;
import java.net.URI;
import java.nio.file.Files;
import java.time.Instant;
import java.time.format.DateTimeFormatter;
import java.util.*;
import java.util.function.*;
/**
* This directly hits Solr's REST API using only native Java. See:
* <p>
* http://lucene.apache.org/solr/guide/7_3/indexing-and-basic-data-operations.html
* <p>
* For more details and more production-ready options.
* <p>
* To run this file:
* <pre>
* java Index.java
* </pre>
* This will print a help message with more usage information.
*/
public class Index
{
private static final Function<Object, String> NOW_AS_ISO;
private static final String INDEX_URL;
private static void printUsage()
{
System.err.print("""
Usage: java [ -DsolrPort=8983 ]
[ -DindexName=documents ]
Index <data-directory>
Options must be before the class file.
Defaults are as listed.
The first argument after the class file must be
the directory data is stored in.
The first level of directories names the MRN,
the second level of directories are the source keys,
and the filename is included as part of the document id.
So radiology files for patient 27-77 would appear like:
27-77/source3/visit1.html
27-77/source3/visit2.html
27-77/source3/report1.html
etc.
(Recall that the radiology source has id "source3".)
Each file can have a related properties file:
27-77/Radiology/visit1.properties
27-77/Radiology/visit2.properties
27-77/Radiology/report1.properties
etc.
which populates additional document fields, such as
document type (DOC_TYPE) or department (DEPT):
DOC_TYPE=Discharge note
DEPT=Internal medicine
""");
System.exit(1);
}
static
{
NOW_AS_ISO = k -> DateTimeFormatter.ISO_INSTANT.format(Instant.now());
var port = Integer.parseInt(System.getProperty("solrPort", "8983"));
var indexName = System.getProperty("indexName", "documents");
INDEX_URL = "http://localhost:" + port + "/solr/" + indexName + "/update/json/docs";
}
public static void main(String[] args)
{
if (args.length != 1)
{
printUsage();
}
try
{
importData(args[0]);
}
catch (Exception e)
{
e.printStackTrace();
System.err.println("\n\nEncountered error!\n\n");
}
}
private static void importData(String baseDir) throws Exception
{
var docProps = new Properties();
File[] patients = new File(baseDir).listFiles(File::isDirectory);
if (patients == null) return;
for (var patient : patients)
{
String mrn = patient.getName();
File[] sources = patient.listFiles(File::isDirectory);
if (sources == null) continue;
for (var source : sources)
{
String sourceKey = source.getName();
File[] docs = source.listFiles(f -> f.isFile() && !f.getName().endsWith("properties"));
if (docs == null) continue;
for (var doc : docs)
{
String docId = mrn + File.separator + sourceKey + File.separator + doc.getName();
loadPropertiesOfDoc(docProps, doc);
docProps.put("ID", docId);
docProps.put("MRN", mrn);
docProps.put("SOURCE", sourceKey);
docProps.put("RPT_TEXT", Files.readString(doc.toPath()));
docProps.computeIfAbsent("ENCOUNTER_DATE", NOW_AS_ISO);
indexDocument(docProps);
}
}
}
}
private static void indexDocument(Map<Object, Object> doc) throws Exception
{
var maxAttempts = 3;
for (var attempts = 0; attempts < maxAttempts; attempts++)
{
var connection = (HttpURLConnection) new URI(INDEX_URL).toURL().openConnection();
connection.setRequestMethod("POST");
connection.setRequestProperty("Content-Type", "application/json");
connection.setDoOutput(true);
writeDocToSolr(doc, connection.getOutputStream());
int status = connection.getResponseCode();
if (status / 100 == 2) return;
else
{
connection.getInputStream().transferTo(System.err);
System.err.printf("Got status %d from Solr: waiting one second to retry\n", status);
System.err.flush();
Thread.sleep(1000);
}
}
System.err.printf(
"Attempted sending %s %d times but failed each time; moving on\n",
doc.get("ID"),
maxAttempts
);
}
private static void loadPropertiesOfDoc(Properties docProps, File doc) throws IOException
{
docProps.clear();
var propFilename = doc.getName();
propFilename = propFilename.substring(0, propFilename.indexOf('.')) + ".properties";
var propertiesFile = new File(doc.getParentFile(), propFilename);
if (propertiesFile.exists()) docProps.load(new FileReader(propertiesFile));
}
private static void writeDocToSolr(
Map<Object, Object> map, OutputStream outputStream
) throws Exception
{
var writer = new OutputStreamWriter(outputStream);
writer.write("{\n");
Iterator<Object> iterator = map.keySet().iterator();
while (iterator.hasNext())
{
var key = (String) iterator.next();
var value = (String) map.get(key);
writer.write(" \"");
writeStringEscaped(key, writer);
writer.write("\": \"");
writeStringEscaped(value, writer);
writer.write('"');
if (iterator.hasNext()) writer.write(',');
writer.write('\n');
}
writer.write("}\n");
}
private static void writeStringEscaped(String s, Writer writer) throws IOException
{
for (int i = 0; i < s.length(); i++)
{
char c = s.charAt(i);
int p = Character.codePointAt(s, i);
if (p <= 31 || c == '"' || c == '\\') writer.write(String.format("\\u%04x", p));
else writer.write(c);
}
}
}
Indexing with Python
Below is a sample script demonstrating how indexing can be done with Python. This is a simple skeleton script showing how to use pyodbc
and pysolr
together to upload notes via a generic database connection into Solr. This script assumes that what is needed to query/stage the data is done in advance on the database side or in a pipeline prior to this code being run. Note that Solr will not accept JDBC connections unless you are running the Solr cloud version which has not been tested with EMERSE.
# Script courtesy of Daniel Harris, University of Kentucky, August 2020
import pyodbc
import pysolr
from requests.auth import HTTPBasicAuth
cnxn = pyodbc.connect('DSN=<DSN>')
cursor = cnxn.cursor()
query = u'''
<query>
'''
try:
solr = pysolr.Solr('<SOLR_URL>:8983/solr/documents',always_commit=True,auth=HTTPBasicAuth('<SOLR USERNAME>','<SOLR PASSWORD>'), verify=False)
with cnxn:
try:
cursor.execute(query)
rows = cursor.fetchall()
for row in rows:
# fields will vary according to your query, replace X with column #
enc_date = row[X].strftime('%Y-%m-%dT%H:%M:%SZ')
update_date = row[X].strftime('%Y-%m-%dT%H:%M:%SZ')
rpt_date = row[X].strftime('%Y-%m-%dT%H:%M:%SZ')
solr.add([
{
"SOURCE": "<INSERT SOURCE ID>",
"ID": row[X],
"RPT_TEXT":row[X],
"MRN":row[X],
"LAST_UPDATED":update_date,
"ENCOUNTER_DATE":enc_date,
"RPT_DATE":rpt_date,
"DOC_TYPE":row[X],
"SRC_SYSTEM":"<SRC SYSTEM LABEL>"
},
])
except Exception as e:
print("Error:")
print(e)
cnxn.close()
except Exception as e:
print(e)
Indexing using Solr DIH (Data Import Handler)
The Data Import Handler has been deprecated and will be removed from the Solr project as of Solr vesion 9.0 It is being moved to a third party plugin. The directions below are being left in this guide for now in case the tool is still useful for important data into EMERSE. |
While the DIH is useful to understand how the indexing process works, the DIH is slow compared to other methods and is not multi-threaded so we do not recommend it for large-scale implementations. The DIH can be configured to access a source system or database, with details about how to retrieve and potentially ‘transform’ the document constructed in SQL. For example, some documents may be in a database with a single document split across multiple rows. The document can be re-assembled into a single text field using SQL and then Solr can process it. Note that Solr expects a document to be ‘pre-assembled’ and does not itself concatenate fields to reconstruct a document from pieces, which is why this should be done in the SQL that retrieves the document. With the DIH you can define the query and the target source in its associated configuration file, and it will pull documents from that source and present them to Solr for indexing.
To get started with DIH, look at the GitHub repository for the project.
Indexing with ETL tools
Documents can be pushed to Solr using ETL tools. In the past, we have used Pentaho Data Integrator (PDI) to do our indexing and other data-pipeline work.
Many possible implementations of PDI jobs could help with indexing documents, but one scenario we have experimented with is where PDI reads a database and for each row does a HTTP POST
call to Solr with the document and metadata in a JSON type of structure.
When using PDI HTTP connectors, consider sending large batches of documents. This will prevent having to open a connection for each document. For further performance improvement, consider enable partitioning in transforms, so that multiple threads are enabled for at least the HTTP portion of this type of PDI job. http://wiki.pentaho.com/display/EAI/Partitioning+data+with+PDI |
About Solr
Updates and Solr Documents
Solr determines if a document should be added versus updated/replaced based on a unique document key. If you send Solr a document with a previously used key, Solr will replace the older version of the document with the newer version, but the document will still exist, flagged as replaced, in the index files. This may be needed in cases where the document was updated (e.g., addended) or even where the metadata has changed (e.g., the document was previously assigned to the wrong patient MRN). Over time, Solr may contain numerous deleted documents that can degrade performance. Solr provides an Optimize command to rewrite the indexes to remove these. For more information see Solr Optimization in the Configuration and Optimization Guide.
Solr Configuration Details
EMERSE requires that several aspects of Solr be configured in a specific way. We recommend not changing these configuration settings since doing so might change the way the system performs. A few of these are explained below. Most details about the various configurable components are not described here, since they are already well described in the official Solr documentation. Many of the configuration details are defined in managed-schema
. In general, changes to the Solr schema should be made through the admin UI. If they are made directly to managed-schema
Solr should not be running or changes could be lost.
Stop Words
Stop words are usually ignored by search engines because they add little meaning to the phrases being searched, and they take up additional storage space. In the case of medical documents, many stop words are legitimate acronyms and abbreviations and, as a result, EMERSE does not use any stop words. Examples of stop words with additional meaning can be seen in the table below. There are tradeoffs with this approach, but by including these additional words it should help to ensure that users will be able to find terms that otherwise might be missed.
Traditional Stop Word | Meaning of Acronym/Abbreviation |
---|---|
a |
apnea |
an |
aconthosis nigricans |
and |
axillary node dissection |
are |
active resistance exercise |
as |
aortic stenosis |
at |
ataxia-telangiectasia |
be |
below elbow |
but |
breakup time |
if |
immunofluorescence |
in |
inch |
is |
incentive spirometry |
it |
intrathecal |
no |
nitric oxide |
on |
one nightly |
or |
operating room |
the |
trans-hiatal esophagectomy |
to |
tracheal occlusion |
was |
Wiskott-Aldrich syndrome |
will |
William (also used in the term 'legal will') |
Analyzers
Analyzers are used within Solr to determine how to process the documents for indexing, as well as processing the query itself. This includes determining how the document or query should be tokenized, and how to deal with case. We define two field 'types'. One is Text_general_htmlstrip
which is used by the RPT_TEXT
field and Text_general_htmlstrip_nolowercase
which is used by the RPT_TEXT_NOIC
field, the latter of which is for keeping documents in a case-sensitive manner (needed for case-sensitive searches to help distinguish common English words such as "all" from acronyms such as "ALL", or acute lymphoblastic leukemia). These should generally not be modified, but we point them out here to highlight configuration settings that could affect search results.
Further, we define a character map in mapping-delimiters.txt
to aid in tokenization and indexing. These can be found below, and essentially replace these characters with a space. This means that phrases such as "17-alpha-hydroxyprogesterone", "O157:H7", and "125.5" will be indexed as separate words: "17", "alpha", "hydroxyprogesterone", "O157", "H7", "125", "5".
"_" => " " "." => " " ":" => " "
This character map also normalizes a few characters to help ensure consistency with searches. This includes mapping curly quotes (single and double) to straight quotes. A similar conversion happens when entering text into the user interface so that all quotes are automatically converted to the plain/straight variety.
Integrating Documents from the Epic EHR
Many academic medical centers use the Epic EHR. There are multiple ways in which to get documents out of Epic, each with its own advantages and disadvantages. Several of these options are described below. Regardless of how the documents are extracted, we recommend storing documents extracted from the EHR in a document repository. Further details about a repository can be found in the EMERSE Architecture Guide.
Regardless of what approach is used for extracting notes, it may be worth concatenating other data to the note to make a larger, more comprehensive, and self-contained note detailing additional aspects of the patient encounter. For example, if a clinical note from an encounters is extracted, it is possible through other queries to extract a medication list at the time of the encounter, append it to the note, and then store this larger, concatenated merged document in a document repository. Such additional data may be useful for users.
It is also possible to incorporate images or drawings within a document before indexing with Solr. Details about how to do this can be found in section on Images in Documents in the Additional Considerations Guide.
Various approach for obtaining and integrating documents from the Epic EHR are described below.
HL7 Interface
The current way in which EMERSE at Michican Medicine receives encounter summaries and notes from Epic is via an Epic Bridges outbound HL7 interface. Details about this interface can be found here: http://open.epic.com/Content/specs/OutgoingDocumentationInterfaceTechnicalSpecification.pdf (Epic account required)
There may be a fee required by Epic to turn on this interface. The emitted HL7 messages include a report, which includes the clinical note as well as other structured data from an encounter in RTF format, which (importantly) preserves the formatting and structure of the notes. The reports are highly configurable by combining Epic print groups, which dictate the content of the report/RTF file. This can make searching more comprehensive for users. The RTF files obtained via this interface are converted to HTML by the commercial software Aspose.Words API, and stored in our document repository for nightly indexing by Solr. For more details, see: RTF to HTML file conversion.
Available metadata in the HL7 message, such as Encounter Date
, Department
, and Edit Date
, are also stored in the repository along with the note. We recommend that you try to only include finalized, or ‘signed’ notes in the repository to prevent the need to continually monitor for document changes and frequent re-processing and re-indexing. However, such decisions will need to be made locally depending on the local use cases, and needs/expectations of users.
One disadvantage of this approach is that it is not useful for loading/extracting historic documents. That is because this outbound document HL7 interface is triggered to send the document based on pre-specified actions (signing a note, for example) and cannot be called on an ad hoc basis to pull historic notes.
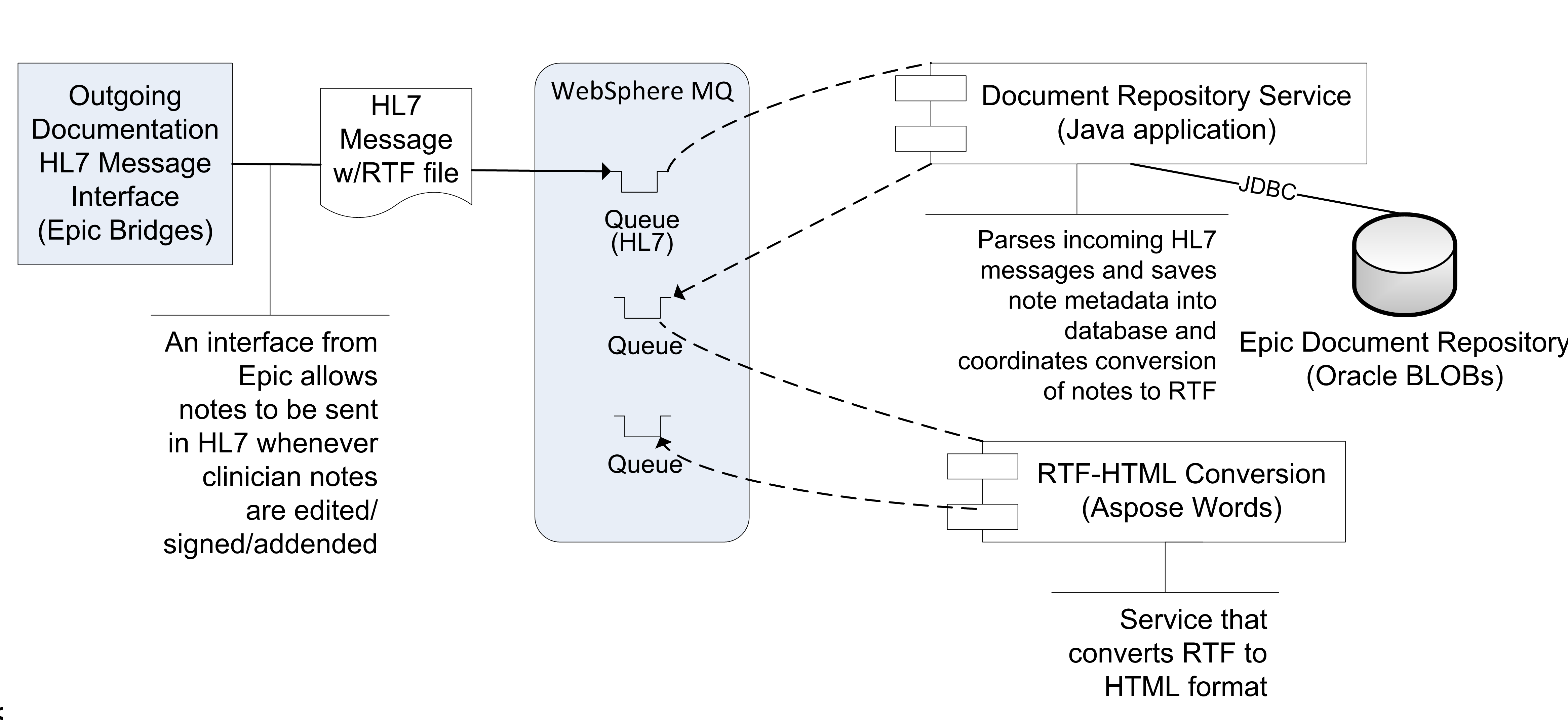
Epic Clarity
It is also possible to receive notes through the Epic Clarity database; however, at the time of this writing, at most institutions Clarity strips a large amount of the document formatting (i.e., basically converting the document from RTF to plain TXT), and thus display of the notes will not look as they appear in Epic. The original document formatting may be essential for understanding/interpreting the document once displayed within EMERSE, including the structure of tables, and other common markup such as bolding, italics, etc. Epic Clarity can preserve some formatting such as line breaks, but doesn’t include tables and other text formatting.
Many Epic instances may be using the default configuration for moving documents from Chronicles to Clarity, and this default configuration will strip out line feeds/carriage returns during the ETL process. However, this can be changed through configuration of the "Character Replacement Settings" which is part of the KB_SQL Generation Settings
. Essentially, the ETL process cannot handle many control characters (such as line feeds), so the character replacement will translate the line feeds to to a different character that the ETL can handle, and then translate them back for storage in Clarity. This translation would have to be turned on for the NOTE_TEXT
field, or something with a similar name.
If there is a desire to use Clarity to obtain notes, we suggest looking into the following tables:
HNO_INFO (note metadata)
HNO_ENC_INFO (notes /encounter linkage)
HNO_NOTE_TEXT (actual note text)
These tables don’t seem to be documented in Epic’s Clarity ambulatory care documentation, even though outpatient notes are stored in the same tables along with the inpatient notes as of Epic 2015. However, The inpatient Clarity “training companion” does have an overview of these tables, and they are mentioned in the Clarity data dictionary, so between these two references you should be able to build a query that collects Epic notes.
Additionally, it should also be possible to move the notes in their original RTF
format from Chronicles to Clarity. This is advantageous since it will help preserve much of the formatting of the original notes (bold text, italics, font sizes, tables, etc). Assuming that the plain text documents would continue to be moved from Chronicles to Clarity (the default configuration for Epic), an additional custom table would have to be created within Clarity to hold the RTF-formatted notes. It will also be necessary to set up the ETL to extract HNO #41
(the location within Chronicles that holds the RTF-formatted notes) directly from the Chronicles database.
When sending these RTF-formatted Clarity documents to EMERSE, the RTF would have to be converted to HTML, but various options are available to do this (see : RTF to HTML file conversion.).
Clarity might still be useful in order to keep track of what documents have been changed (for example, a note might be deleted or addended weeks after it has been stored and indexed in EMERSE). |
Storing notes as RTF is advantageous to preserve formatting, but it will increase the storage requirements. We estimate that our RTF documents each take up about 22k of space, although each document does have additional data appended to each one, including the medication list and problem list at the time of the encounter. Further, we estimate that storage for the raw RTF might take up about 10% more space than their plain text (RTF-stripped) counterparts. As of 2021 we began to incorporate images into our documents (encoded in Base64) which further increased the size of the stored documents. |
An interesting story about naming conventions: The name HNO for the note table in Epic came from the original phrase "Shared NOtes", but since HNO was already being used by the system, the second letter (H) in sHared was used instead. |
Epic Web Services
It is possible to build a web service to extract documents that preserves the original formatting of the notes.
At the University of Michigan we have built a custom web service that extracts formatted notes directly from Chronicles (not Clarity). These notes still have their native formatting which is in RTF, but they are converted to HTML and embedded in a SOAP message during the web service call. This approach will require individuals to have experience with writing custom web services and Epic Cache code. Those interested in exploring this option can contact us at the University of Michigan and we can share the Cache code and the web service definition.
As of version Epic 2017, there are two 'out of the box' web services that can be used for extracting notes:
-
GetClinicalNotes
-
GetClinicalNoteDetails
Further information about these web services can be found within the Epic documentation.
Epic’s webservices are not used for EMERSE at Michican Medicine, but are being used elsewhere in our health system. The major challenge with using the webservices is that they require knowledge of the primary note identifier before making the webservice call. It should be possible, however, to use clarity to pull note identifiers and then make a webservice call to retrieve the note as RTF/html. Other approaches may work equally as well. |
Depending on the integration approach, it may be useful to setup a staging database that sits between the source systems and the EMERSE Solr indexes. The primary use of this at Michigan Medicine is to store the documents as they are received from Epic as HL7 messages. They are not directly stored in Solr, even though Solr ultimately creates its own local copy once a documents is sent from the staging database to Solr. |
Integrating Documents from EHRs more generally
Fast Healthcare Interoperability Resources (FHIR)
FHIR is an emerging standard that is meant to be EHR vendor-independent so that it can work across all EHR systems that support the standard. Using FHIR could potentially be a viable approach for nearly any modern EHR. With support from the NCI Informatics Technology for Cancer Research (ITCR), a team at the University of Utah developed a FHIR-based tool for bulk extracting documents in their native format based on an input set of medical record numbers (MRNs). The University of Michigan further modified the code, using FHIR R4, whereas the original Utah-developed code used STU3. These projects/code can be found on our EMERSE GitHub repository (contact us for access).
The University of Michigan has tested this software against a "support" Epic environment and not a true production environment. Nevertheless, our results should provide a guidepost for assessing whether this tool is appropriate for your use.
Through a collaboration with the University of Utah, we are able to provide a FHIR-based tool for bulk-extracting documents from EHRs. A description of our findings is below, so that it may serve as a useful guide for others considering using this tool.
-
With Epic, the software pulls documents directly from Chronicles, the transactional database used for active patient care (with other EHR systems it is unclear where the data would be pulled from since it will depend entirely on the vendor’s implementation of the standard). Because the code extracts data directly from the transactional system, it would be safe to assume that some limitations would be place on usage by each institution, especially during peak business hours. At the time of this writing (2022) Epic does not have a way to throttle usage.
-
The data are extracted in HTML format and contain the native formatting of the documents (e.g., bold, italics, tables, etc) which is ideal for viewing within EMERSE.
-
We have found that some images, such as those within educational materials are embedded within the HTML using Base64 encoding, but the patient-specific clinical images are not embedded.
-
FHIR is not designed to pull large amounts of information at one time. In our performance testing on a staging environment, we found the document extraction rate to be approximately 3 documents per second.
-
The only filter currently supported by the code is a start date (thus the date range would be the start date through the current date at the time of code execution). There is not a way to request specific document types, or to request metadata. Thus, if a document is edited, it may not be eay to identify documents that have been updated but were previously extraced.
-
Fo Epic systems, it is possible to submit a medical record number as an identifier using “identifier=MRN|000000000” as the request parameter on the FHIR interface directly to query patients. This is an extension from Epic implementation of FHIR. Then the FHIR identifier is retrieved from the result of the patient query. For all following queries like DocumentReference, Binary etc. the FHIR ID will be used.
We would like to acknowledge the NCI ITCR program (Grants 5U24CA204800 and 5U24CA204863) for supporting development of this tool, as well as the team at the University of Utah including: Douglas Martin, Guilherme Del Fiol, and Kensaku Kawamoto. |
Patient Integration
EMERSE requires that all patients referenced in documents exist in the Patient database table.
At the University of Michigan, the Patient table is updated once per day (overnight) which coincides with the timing of indexing all new/changed notes (also once per day). The Solr indexing process does not require that this table be up to date, but the EMERSE system will need the MRNs to match between the PATIENT
database table and the Solr indexes for everything to work properly when users are on EMERSE.
Patient data should be loaded into the Patient database table, and then these data get copied over to a Solr index created to match the patient data with the search results. This copying process is handled by Solr. The default settings for when this copying occurs is configurable, which is detailed in the Configuration Guide.
PATIENT table
Table: PATIENT
Population: From external source (such as EHR, or a staging document repository)
Population Frequency: Can be variable, but once per day is reasonable
The EMERSE schema includes a patient table with medical record number (MRN), name, date of birth, and other demographic information which is displayed in the search results. Data in this table are used to display the patient name, validate user-entered or uploaded MRNs and to calculate current ages of the patients. Other demographic data are used to summarize the populations found in a search.
Although the coded demographics information is not required, some features such as the demographics breakdowns within the All Patients search feature will not work if sex, race, ethnicity are not populated. Further, EMERSE can filter the results based on these demographics data. |
For all documents indexed, there must be a corresponding patient in the patient table with a medical record number (MRN) that matches the document. This should be taken into consideration when determining the frequency for updating this table. |
Column name | Description | Required or Optional |
---|---|---|
id |
Primary Key |
Required |
external_id |
Medical Record Number |
Required |
first_name |
First Name |
Required |
middle_name |
Middle Name |
Optional |
last_name |
Last Name |
Required |
birth_date |
Birth Date — used to calculate current age |
Required |
sex_cd |
Sex |
Optional (but highly recommended) |
language_cd |
Language (Currently not used. Can be populated in the system, and used for some advanced filtering, but will not be displayed to users.) |
Optional |
race_cd |
Race |
Optional (but highly recommended) |
marital_status_cd |
Marital Status (Currently not used. Can be populated in the system, and used for some advanced filtering, but will not be displayed to users.) |
Optional |
religion_cd |
Religion (Currently not used. Can be populated in the system, and used for some advanced filtering, but will not be displayed to users.) |
Optional |
zip_cd |
ZIP code |
Optional |
create_date |
Date the row was created. Can be used to track changes to the table. |
Optional |
update_date |
Date the row was updated. Can be used to track changes to the table. |
Optional |
ethnicity_cd |
Ethnicity |
Optional (but highly recommended) |
deleted_flag |
Logical delete flag. Useful for merged patient MRNs that result in obsolete MRNs. (see: Merges/Splits) Valid values are 1 = yes, deleted; 0 = no, not deleted |
Required |
deceased_flag |
Currently not used. Valid values are: 1 = yes, deceased; or 0 = no, not deceased |
Required |
updated_by |
To keep track of 'updated by' details |
Optional |
created_by |
To keep track of 'created by' details |
Optional |
Obsolete/outdated MRNs should never be deleted from the table. Rather, they must remain and be flagged with the deleted_flag . Otherwise the functionality to warn users about obsolete MRNs will not work. Further, if the MRN is simply updated in the table rather than marking the older MRN as deleted and adding a new row, users will not be warned that the MRN has changed. This could cause issues if the MRNs are also stored in other data capture systems since the user would not know that the MRN in the external system is no longer valid.
|
The data in the Patient table are automatically copied to a Solr index, by default once per day. This is detailed in the Configuration Guide.
|
Research Study Integration
EMERSE is often used to aid in research studies. While not required, there is a provision for users conducting research to select a research study they are associated with at the "Attestation Page" after successfully logging in. EMERSE then links that particular session with the study in the EMERSE audit logs. Loading your institutional IRB data into the research tables in EMERSE will enable it to show the studies that each user is associated with, that are not expired, and that have a valid status (e.g., Approved status).
At the University of Michigan we use a commercial IRB tracking system (Click Commerce). A subset of the data from this system are moved to a data warehouse every night, and we extract a subset of data from that warehouse to bring into EMERSE for validation of users to studies. A Pentaho Data Integrator job, external to EMERSE, copies this subset to the research study tables in EMERSE, nightly.
If there are large delays in updating the RESEARCH_STUDY tables, it can delay users access to EMERSE, since the study will not appear in the attestation screen for the user to select. There is also a chance that a user could still be permitted to be approved for use on a study even if the study was revoked (again because of the delay introduced by nightly refreshes). |
Research Studies and Attestation
Immediately after each login, every user is required to ‘attest’ to their use of EMERSE for that session by specifying their reason for using the system. This is called the Attestation page, and the results are stored in the SESSION_ATTESTATION table. EMERSE provides three basic options (configurable by a system administrator; these options can be disabled) for this attestation: (1) a Free Text field, (2) pre-populated Common Use Cases (for example, “Quality Improvement”, “Patient Care”, “Infection Control”, etc), and (3) a list of research studies to which a user is associated. Additional tables RESEARCH_STUDY_ATTESTATION
, and ATTESTION_OTHER
may contain additional information depending on whether the user specifies a research study, or other reasons. The free text option would be used by users when no other attestation choices are reasonable. Additionally, previously used entries from the free text box will appear in the table, along with any IRB-approved studies, for the user’s convenience.
For our implementation at Michigan Medicine, we pull data on all studies in the IRB system, even if the study/person is not currently a part of EMERSE. This is because the dataset is generally small, and it makes it easier for users to validate their studies if the data are already populated, once the user is given an EMERSE account |
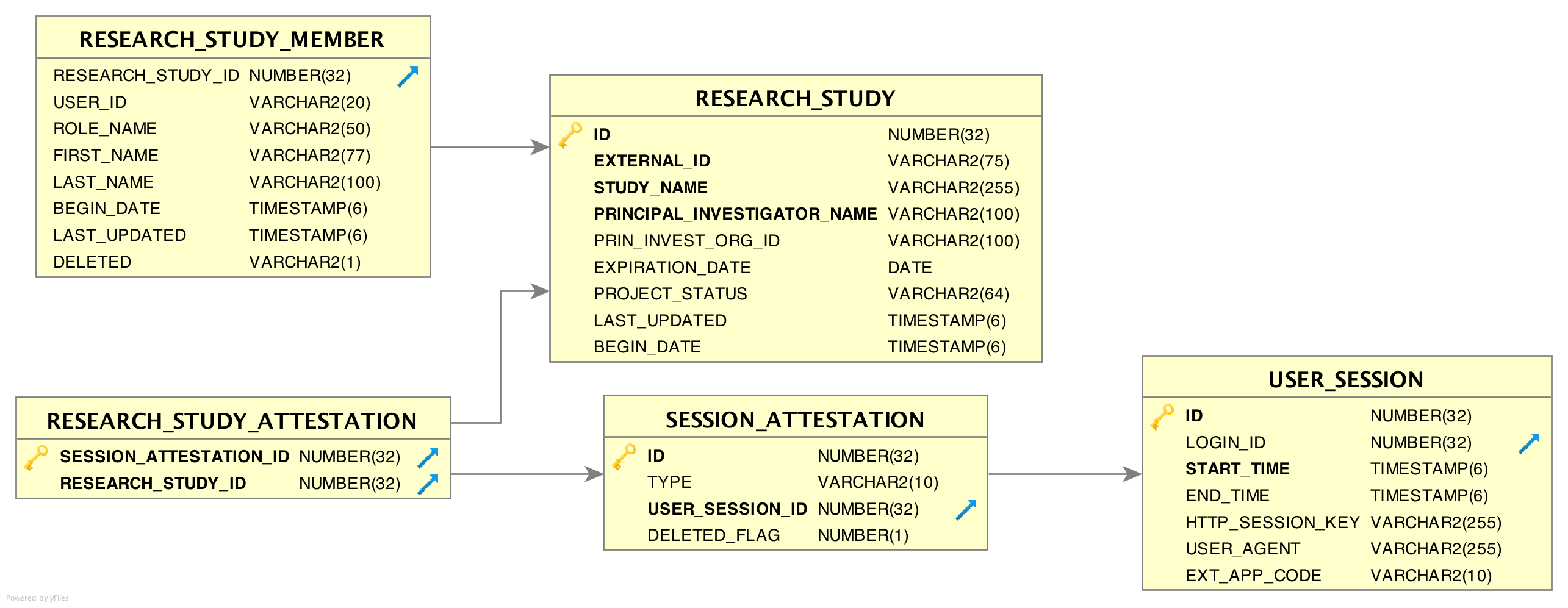
RESEARCH_STUDY table
Table: RESEARCH_STUDY
Population: Populated from external source such as an electronic IRB system
Population Frequency: Can be variable, but once per day is reasonable
If a user is required to select his/her study from the table, then delays in moving IRB data to EMERSE after IRB approval can result in delays access for that user. |
This table contains information about research studies. Using this table and RESEARCH_STUDY_MEMBER
allows EMERSE to show a list of studies the end user is associated with.
Column name | Description | Required or Optional |
---|---|---|
id |
Primary Key |
Required |
external_id |
IRB study number — used to link specific studies to usage, and is very helpful for tracking research usage |
Required |
study_name |
Name of the study |
Required |
principal_investigator_name |
Name of the principal investigator |
Required |
prin_invest_org_id |
id of principal investigator. Not currently used by EMERSE. This could be a user id, or email, but it is a good idea to ensure it is unique. |
Optional |
expiration_date |
Expiration date of study. Used to determine if a user should be allowed to proceed. If the expiration date is older than the current date, the user will not be able to select it in the attestation GUI. |
Required |
project_status |
Current project status. This is used to track where a study is in the review and approval process. Only certain study statuses allow access to EMERSE for research. The statuses that allow a study to be selected during attestation are defined in the |
Required |
last_updated |
A last updated date is not used by EMERSE, but can be useful for troubleshooting and tracking changes to the table. |
Optional |
begin_date |
This originally referred to the date the study began or should be allowed to begin. This field that can be used for tracking and troubleshooting. |
Optional |
VALID_RES_STUDY_STATUS table
Table: VALID_RES_STUDY_STATUS
Population: By System Admin.
Population Frequency: May only need to be done once, at the time of system setup. May need periodic updates if the source data (such as from IRB system) defining study status is changed.
EMERSE contains a simple table defining study statuses. The statuses that are initially populated in the system (loaded up in the build script) are unique to Michigan Medicine—that is, they were developed locally and are implemented in our separate electronic IRB tracking system—and other implementations would have to have their own set of valid statuses if these were to be used to validate and approve usage for research. If the status of a research study is not in this table, EMERSE will not allow the study to be used for attestation; in other words, the study would not even be displayed to the user to select.
Column name | Description | Required or Optional |
---|---|---|
status |
A list of study statuses that EMERSE considers valid in terms of allowing a user to proceed. These statuses are generally defined by the IRB and are universal across studies. |
Required |
VALID_RES_STUDY_STATUS
Table Example:
Status |
---|
Exempt Approved - Inital |
Approved |
Not Regulated |
Exempt Approved - Tranistional |
RESEARCH_STUDY_MEMBER table
Table: RESEARCH_STUDY_MEMBER
Population: Populated from external source such as an electronic IRB system
Population Frequency: Can be variable, but once per day is reasonable
This table contains information about study team members, and is related to the RESEARCH_STUDY
table, described above. Each study can have one or many study team members.
This table at Michigan Medicine contains information on all study team members for all studies, whether they have an EMERSE account or not. |
Column name | Description | Required or Optional |
---|---|---|
RESEARCH_STUDY_ID |
Foreign key reference to row |
Required |
USER_ID |
Foreign key reference to row in |
Required |
ROLE_NAME |
A string describing a person’s role on the study team. EG. “PI”, “Staff”, “Study Coordinator”. This can be useful when generating usage reports. |
Optional |
FIRST_NAME |
First name of the user who is on the study. It would likely be populated from the source IRB system, but it is not used at all by EMERSE. Nevertheless, it may be useful when generating reports. |
Optional |
LAST_NAME |
Last name of the user who is on the study. It would likely be populated from the source IRB system, but it is not used at all by EMERSE. Nevertheless, it may be useful when generating reports. |
Optional |
BEGIN_DATE |
This is not currently used by EMERSE. |
Optional |
LAST_UPDATED |
Date row was last updated |
Optional |
DELETED |
Flag to indicate if the record has been logically deleted. |
Required |
SESSION_ATTESTATION table
Table: SESSION_ATTESTATION
Population: Used internally by EMERSE
Population Frequency: In real time by EMERSE
Each time a user attests to why they are using EMERSE, a row is inserted into this table, which is one of the audit tables. Attestations related to research can be joined to the RESEARCH_ATTESTION
table. Non-research uses can be joined to ATTESTION_OTHER
.
Column name | Description | Required or Optional |
---|---|---|
id |
Primary Key |
N/A (populated internally by EMERSE) |
type |
A string indicating the top level category of attestation. |
N/A (populated internally by EMERSE) |
User_session_id |
A foreign key reference to the |
N/A (populated internally by EMERSE) |
OTHER_ATTESTATION_REASON table
Table: OTHER_ATTESTATION_REASON
Population: By System Admin. Used if Common Use Cases for using EMERSE are desired to ensure consistency of attestation selections.
Population Frequency: May only need to be done once, at the time of system setup.
For non-research attestations, there is a lookup table called OTHER_ATTESTATION_REASON
that lists available options. These can be configured by each institution, and may include commonly used access reasons that don’t involve research (such as quality improvement, patient care, etc). These options provide a simple way for a user to click on one of the pre-populated common reasons for use.
Column name | Description | Required or Optional |
---|---|---|
USER_KEY |
Text based primary key of this table. The column name might better be thought of as as 'reason key'. |
Required |
DESCRIPTION |
The text description that will be displayed in the table of options on the Attestation page. |
Required |
DELETED FLAG |
Has this reason been deleted? (0 = no; 1= yes) |
Required |
DISPLAY_ORDER |
Order of display in the UI. Can be any integer, but should be unique per row. The buttons are ordered by this column via sql sort. Generally start with 0,1,2, etc. This is no longer used as of version 6.0 |
Optional. This is no longer used as of version 6.0 |
OTHER_ATTESTATION_REASON
Table Example:
USER_KEY | DESCRIPTION | DELETED_FLAG | DISPLAY_ORDER * |
---|---|---|---|
QI |
Quality Improvement |
0 |
0 |
RVPREPRES |
Review Preparatory to Research |
0 |
1 |
STDYDESC |
Study involving only decedents (deceased patients) |
0 |
2 |
* This column is no longer used as of version 6.0.
ATTESTATION_OTHER table
Table: ATTESTATION_OTHER
Population: Used internally by EMERSE
Population Frequency: Application dependent
The free text reasons that users enter are stored in a table called ATTESTATION_OTHER
. This is populated by EMERSE and is not customizable by users.
Column name | Description | Required or Optional |
---|---|---|
SESSION_ATTESTATION_ID |
A unique ID for the session attestation. Used for audit logging. |
Required |
FREE_TEXT_REASON |
The free text reason that a user entered. |
Required |
OTHER_ATTEST_REASON_KEY |
This will currently only be populated by the system with |
Required |
ATTESTATION_OTHER
Table Example:
SESSION_ATTESTATION_ID | FREE_TEXT_REASON | OTHER_ATTEST_REASON_KEY |
---|---|---|
50208 |
Testing out the system |
FRETXT |
52060 |
Testing out the system |
FRETXT |
46051 |
Looking up a patient in clinic |
FRETXT |
71052 |
infection control monitoring |
FRETXT |
74107 |
cancer registry operational work |
FRETXT |
LDAP integration
EMERSE can be configured to use LDAP for user authentication. At this time, however, EMERSE authorization can not be done exclusively with LDAP - only users in the LOGIN_ACCOUNT table can access EMERSE even when LDAP is enabled. This was done intentionally so that accounts could be provisioned/revoked on a manual basis, pending review. The Configuration Guide provides details on changing settings that will enable LDAP authentication.